With the continuing decrease in computational cost, machine learning has made its way into more and more applications. The driving factor for successful machine learning is the broad availability of well-described high-quality data. This is one of many factors why data has been described as the new oil of the digital economy.
Within CRC 1333 "Molecular Heterogeneous Catalysis in Confined Geometries", we apply the FAIR data principles (findable, accessible, interoperable, reusable) by establishing data management workflows in various fields of chemistry such as catalysis, organic synthesis, material sciences, analytics, and computational chemistry. Drawing from our experience with the development of our standardised data exchange format EnzymeML we aim to develop and implement useful, novel, bottom-up solutions for research data management. We collaborate closely with the Cluster of Excellence SimTech and the NFDI consortia NFDI4Chem and NFDI4Cat.
Publications
- Pleiss, J.: FAIR Data and Software: Improving Efficiency and Quality of Biocatalytic Science. ACS Catal. 14, 2709--2718 (2024). https://doi.org/10.1021/acscatal.3c06337.
- Giess, T., Itzigehl, S., Range, J., Schömig, R., Bruckner, J.R., Pleiss, J.: FAIR and scalable management of small-angle X-ray scattering data. Journal of Applied Crystallography. 56, 565–575 (2023). https://doi.org/10.1107/S1600576723001577.
Members
Max Häußler
Bioinformatics
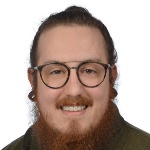
Torsten Giess
Bioinformatics